Unconventional Techniques for Better Insights from Satisfaction Surveys
“We want to hear from you.”
“How did we do?”
“Tell us how we’re doing.”
“How was your experience?”
Every week, it seems, we get a new batch of feedback surveys. Organizations want to know how we feel about the interactions we had with their products and services. They ask for “Just a quick moment of your time” to fill out a “brief” survey about the experience.
Until recently, design teams haven’t shown much interest in these types of surveys, as they mostly have been about marketing the product, not designing it. But, organizational interest in initiatives, such as Voice of the Customer, Customer Experience (CX), and now Customer Centricity has pushed design teams to pay more attention.
The interest comes with great intentions. The people behind the surveys earnestly want to know if the organization has lived up to its promises. They seek any information that can help them improve their designs.
Yet, the data they collect and the way they collect it may not give them what they’re hoping for. In the best case, surveys are seen for what they are and not given much credence within the organization. In the worst cases, serious and expensive decisions are made based on survey data, which drives the organization unknowingly in wrong directions. It’s important that we understand the challenges this data presents, how we can make the best use of it, and how to change what we collect to improve our data quality.
The difference between subjective and objective data.
At a recent conference, I heard a speaker explain to the audience that qualitative data (data collected through methods such as usability testing) was always subjective while quantitative (data from surveys) was always objective. Unfortunately, this commonly held belief isn’t quite true.
In a usability test, the observers might notice that a user was presented a particular error message four times. We’d call that objective data, because it doesn’t matter which observer collected it. Each observer would come to the same conclusion about how many times the error message showed up. There’d be no debate.
However, in that same test, the observers might come to differing conclusions of the user’s frustration level from the repeated error message. We’d call that data subjective, because there’s no objective criteria for establishing a sense of user’s frustration.
The session moderator could ask each participant to say out loud how frustrated they were, for example, on a scale of 1 to 5. Even though each observer would hear a participant say the number four, we’d still call that subjective data, because there’s no objective criteria that every participant uses. The next participant could also say “Four” even though they are frustrated for entirely different reasons.
What separates objective data from subjective data is whether we have an unambiguous set of criteria that allows everyone to classify data similarly. When watching someone buy a product online, we can usually all agree with the price they paid, because that number was clearly displayed on the screen and charged in the transaction.
However, we have no standard way of telling whether the shopping experience was pleasant or aggravating. That’s subjective.
Subjective data isn’t bad. But, we have to see it for what it really is: an opinion about what’s happened. It’s clearly not factual, unlike objective data. For decision making, that distinction makes a difference.
Do you “Like” doing business with us?
In essence, the organizations sending us those feedback surveys are trying to learn if we liked interacting with their products or services. If we did, might we want to do it again? If we didn’t, how might they improve the experience?
There are many different ways to ask if we liked something. Of course, they can ask a simple question like how did we do? Often times, they’ll choose more institution-sounding language, like how satisfied were you with your shopping experience?The worst is when they pick convoluted questions, like Net Promoter Score’s how likely are you to recommend us to a friend or colleague?
No matter how the question is asked, the folks asking are looking for essentially the same answer: did you like the interaction with us? Whether it’s on a 10-point (or in the case of NPS, 11-point) scale or just a yes-or-no answer, the idea is the same. Do our customers like doing business with us?
“Like,” by itself, is not helpful.
The problem is, it isn’t helpful just knowing if customers like us or not. It doesn’t tell us what to do differently. If everyone likes us, we don’t know what they like about us. If we make changes going forward, we might wreck the thing they really like about us.
If everyone tells us they don’t like us, we don’t know what our users are struggling with. We don’t know how to make their experience better. Without more information we’re at a loss on what to change and what not to change.
Putting the data on a scale doesn’t help. It only muddles the data. If, on a scale of -5 (don’t like) to +5 (like), we get an average score of -3.7, then we only know -3.7 is slightly more positive than an average score of -4.2. We still don’t have any data that tells us how to improve our average score.
And an average score is aggregated. When we don’t understand the individual scores, aggregated scores become just aggregated nonsense.
Aggregated scores do not speak to individual differences. There might be totally different underlying reasons why two customers didn’t like dealing with us. Numbers can’t give us any sense of that.
The benefit of matching up behavioral data.
What if we combine the question “whether a user likes us or not” with the data we have on how they use our product or service?” (We don’t want to ask the user if they’ve used it—that’s still subjective—instead use built-in analytics of actual usage data.) For example, an airline could match up customers’ opinions of the airline’s service with whether those customers have flown on any recent flights.
We could segment these two data elements into four groups:
Customers who’ve used the product and say they like us. These customers are interesting because using the product seems to make them happy. Conducting further research to learn what about the product makes them happy could help us explain the benefits to others.
Customers who’ve used the product but say they don’t like us. These might be customers who will switch to a competitor if we don’t fix whatever it is that they don’t like about us.
Customers who don’t use the product, but say they like us. Hmmm. There’s something about the potential of our product that these folks like. Further research might explain what’s preventing them from actually taking advantage of our offerings.
Customers who don’t use the product, and say they don’t like us. These customers have formed their impressions of us from something, but recent use isn’t it. What might it take to get them to try us? Would that make a difference in how they feel about us?
Segmenting this data is useful, but it usually results in us asking more questions than providing actionable answers. Only knowing if someone likes us and if they’ve used us doesn’t help us plan improvements.
Measuring the disappointment of loss.
Recently, we’ve come across a couple of exciting enhanced approaches to subjective satisfaction data. The first one comes from investor Sean Ellis and it’s what he calls his Product-Market Fit question.
Sean uses this question to measure online services that people might use frequently. It is a single question with four answers.
How disappointed would you be if <service> was no longer available?
- I’d be very disappointed.
- I’d be somewhat disappointed
- Meh. It wouldn’t bother me.
- Doesn’t matter. I’m don’t use <service>.
Sean says the people who answer A (very disappointed the service would no longer be available) are the group of most engaged customers. When you have a majority percentage of these, you’ve achieved what investors call “product-market fit.” The goal is to grow the people in Group A by making the service something they feel they’d rather not live without.
Sean’s use of four choices is an interesting alternative to a numeric scale. While we still don’t know why someone feels a certain way when they choose very disappointed versus only somewhat disappointed, we have a clearer way to talk about the findings. More research could reveal the underlying reasons behind each customer’s feelings, which could point the way to improvements.
Enhancing the question by collecting more data.
Rahul Vohra, CEO of email product Superhuman, took Sean’s question and enhanced it to give his team more information to work with. His enhancement is a short, four-question survey that starts with Sean’s disappointment question:
- How disappointed would you be if you could no longer use Superhuman?
This question has the same answers as Sean’s. Rahul then added three open-ended qualitative questions:
- What type of people do you think would most benefit from Superhuman?
- What is the main benefit you receive from Superhuman?
- How can we improve Superhuman for you?
Rahul’s additional three questions add more depth to Sean’s original question. Rahul segments the answers he gets from the respondent’s first answer to Sean’s question about disappointment.
People who said they’d be very disappointed if Superhuman disappeared are Group A folks. Their answer to the type of people who benefit is basically a description of themselves. Their description of the main benefit is the most compelling aspect of the product, telling Rahul what he needs to protect. The Group A respondents’ list of improvements is a nice-to-have list, but not necessary to keep the customer subscribed, as they’re already highly engaged with the product.
Rahul turns his focus to the folks who are in Group B by answering Sean’s question that they’d be somewhat disappointed if Superhuman was no longer available. When the Group B respondents describe the people who most benefit, they’re describing someone slightly different from themselves. Comparing the Group B answers to the Group A answers, Rahul sees who feels the product is not quite for them.
Similarly, when the Group B respondents talk about their main benefit, they’re talking about the things that most make a difference to them. And looking at the Group B list of improvements gives a clear shopping list for shifting these folks into Group A in the future.
Rahul looks for patterns amongst to the Group B respondents to learn which features to invest in. He can look for changes in responses over time, to see if what people are asking for is shifting. This is much more valuable than just having a numeric score from an average satisfaction number.
Observation is still the best way to learn.
There are improvements we can make to subjective satisfaction scores, like shifting towards something akin to Sean and Rahul’s approaches. However, we’ll always be limited by trying to do everything through surveys.
Observation gives us a chance to collect more objective data. We can see how users interact with our designs. We can match what we see directly against the subjective data we’ve collected.
When users say they don’t like something, we can ask them to show us what they don’t like about it, producing richer insights.
We’ll always get our biggest improvement by spending more time directly talking to our customers and observing how they use our products and services. This can’t be done by just a small group of researchers. The entire team, including the folks putting out surveys, need to be exposed directly to customers and users.
Imagine having the kind of data Rahul’s survey reveals alongside direct visits with those respondents. Teams could then regularly visit a dozen or so customers and look specifically for valuable subjective answers, uncovering richer insights. These insights then lead directly to solid data and could provide your design teams the answers they need to drive the delivery of better-designed products and services.
After all, that’s what we’re collecting the data for in the first place.
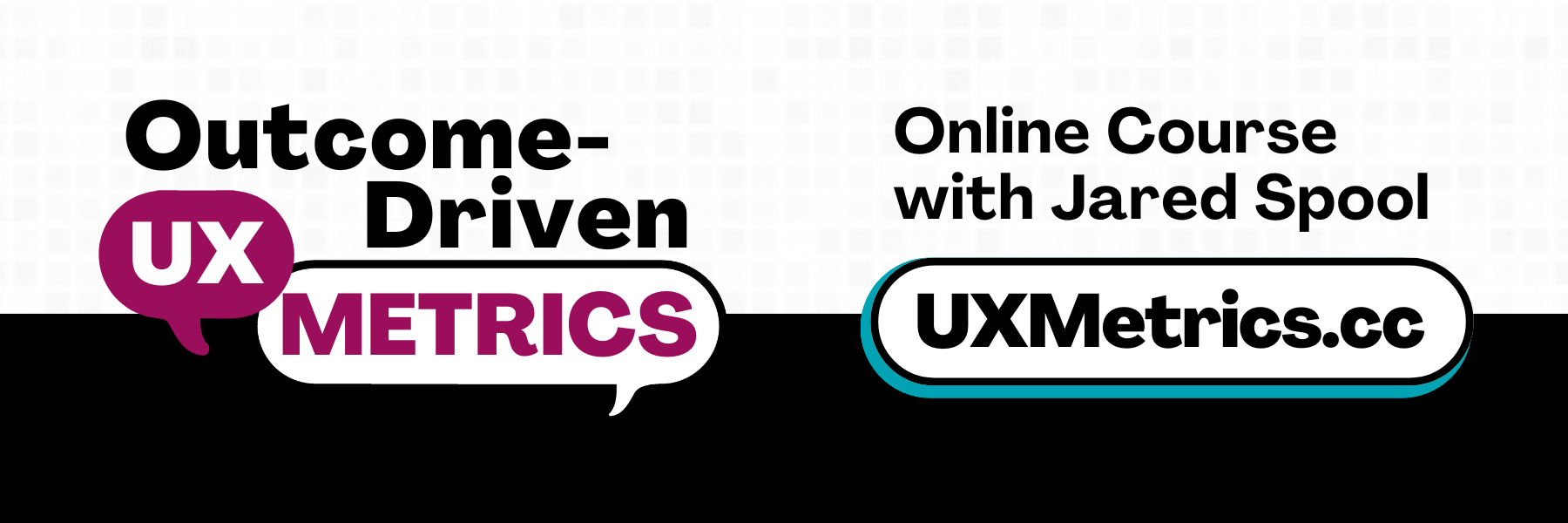
Enroll in Our Four-Week Live Course on Outcome-Driven UX Metrics.
Establish your team’s 2025 UX metrics and goals by investing just 4 hours a week in our new Outcome-Driven UX Metrics course, featuring 8 hours of pre-recorded lectures and 8 hours of live coaching sessions with Jared.
You’ll learn to set inspiring UX goals, boost your team’s strategic impact, and receive personalized coaching, all while gaining access to a community of 51,000+ UX leaders.